UROP Project
differential equations, data science, numerical analysis
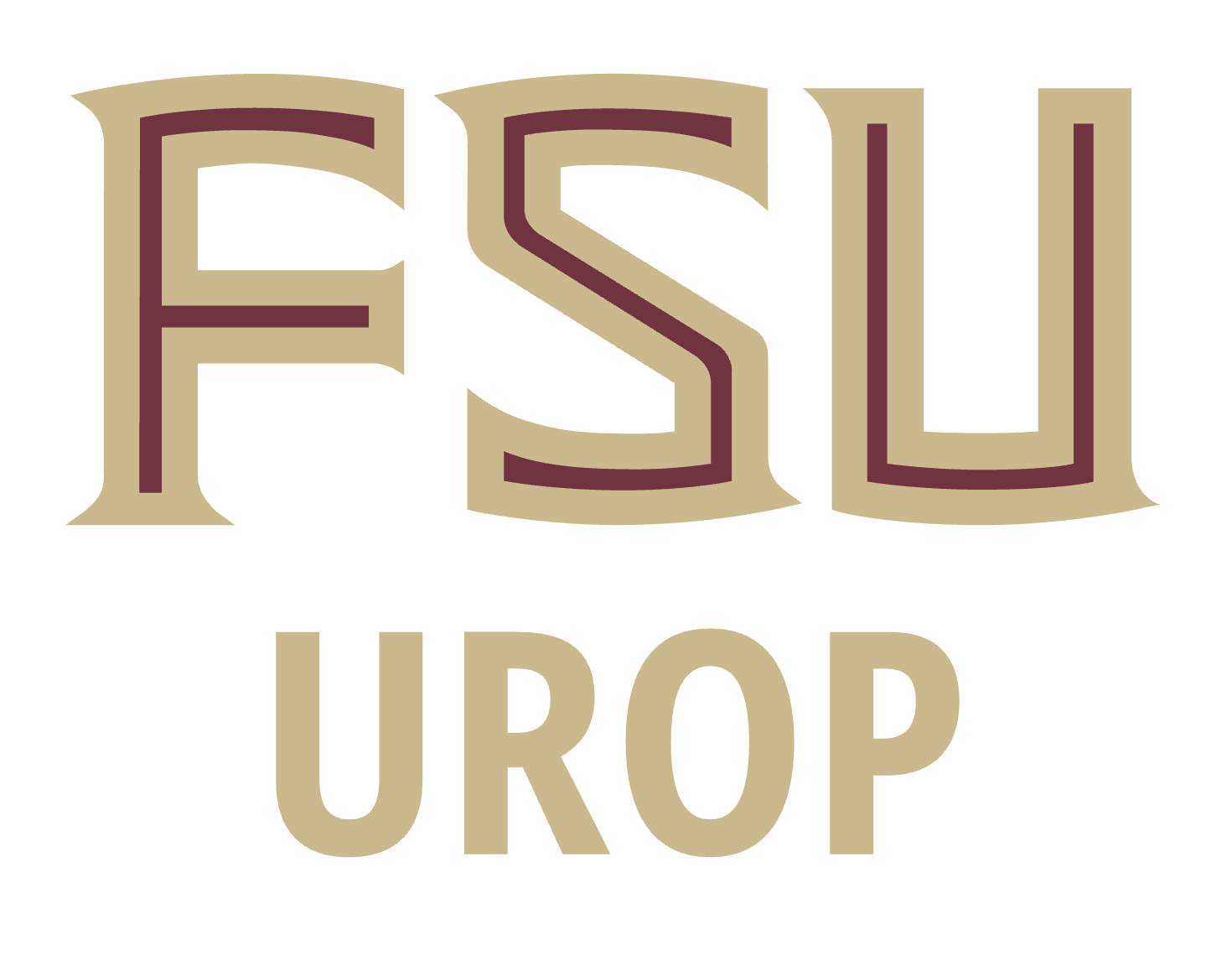
Research Mentor: Mark Sussman,
Department, College, Affiliation: mathematics, Arts and Sciences
Contact Email: sussman@math.fsu.edu
Research Assistant Supervisor (if different from mentor):
Research Assistant Supervisor Email:
Faculty Collaborators:
Faculty Collaborators Email:
Department, College, Affiliation: mathematics, Arts and Sciences
Contact Email: sussman@math.fsu.edu
Research Assistant Supervisor (if different from mentor):
Research Assistant Supervisor Email:
Faculty Collaborators:
Faculty Collaborators Email:
Looking for Research Assistants: Yes
Number of Research Assistants: 2
Relevant Majors: open to all majors
Project Location: On FSU Main Campus
Research Assistant Transportation Required: Remote or In-person: In-person
Approximate Weekly Hours: 10 hours,
Roundtable Times and Zoom Link: Not participating in the Roundtable
Number of Research Assistants: 2
Relevant Majors: open to all majors
Project Location: On FSU Main Campus
Research Assistant Transportation Required: Remote or In-person: In-person
Approximate Weekly Hours: 10 hours,
Roundtable Times and Zoom Link: Not participating in the Roundtable
Project Description
There have recently been many new developments in solving differential equations using data science techniques. See for example the "PINN" ("Physics Informed Neural Networks") method developed by Prof. Karniadakis at Brown University. Are these methods better than classical methods (finite element, finite difference, finite volume, or Runge Kutta methods) for solving differential equations? It could be that the new ``data science motivated'' methods are a transformative development since the methods are "embarrassingly vectorizable" (for GPU processing) and have anecdotally been shown to represent solutions using a minimum number of degrees of freedom. There are many test problems to try and compare classical methods to data science techniques, all ranging in level of difficulty.Research Tasks: Research tasks:
1. develop a classical numerical method (your choice) for solving an ODE or PDE. Computer language is flexible.
2. develop a data science numerical method for solving the same ODE or PDE as in step 1.
3. Compare (1) to (2) in terms of (i) ease of implementation, (ii) accuracy, and (iii) efficiency.
Skills that research assistant(s) may need: required:
basic programming skills. Calculus II or higher.