Research Symposium
25th annual Undergraduate Research Symposium, April 1, 2025
Samir Kanbar Poster Session 3: 1:45 pm - 2:45 pm/ Poster #233
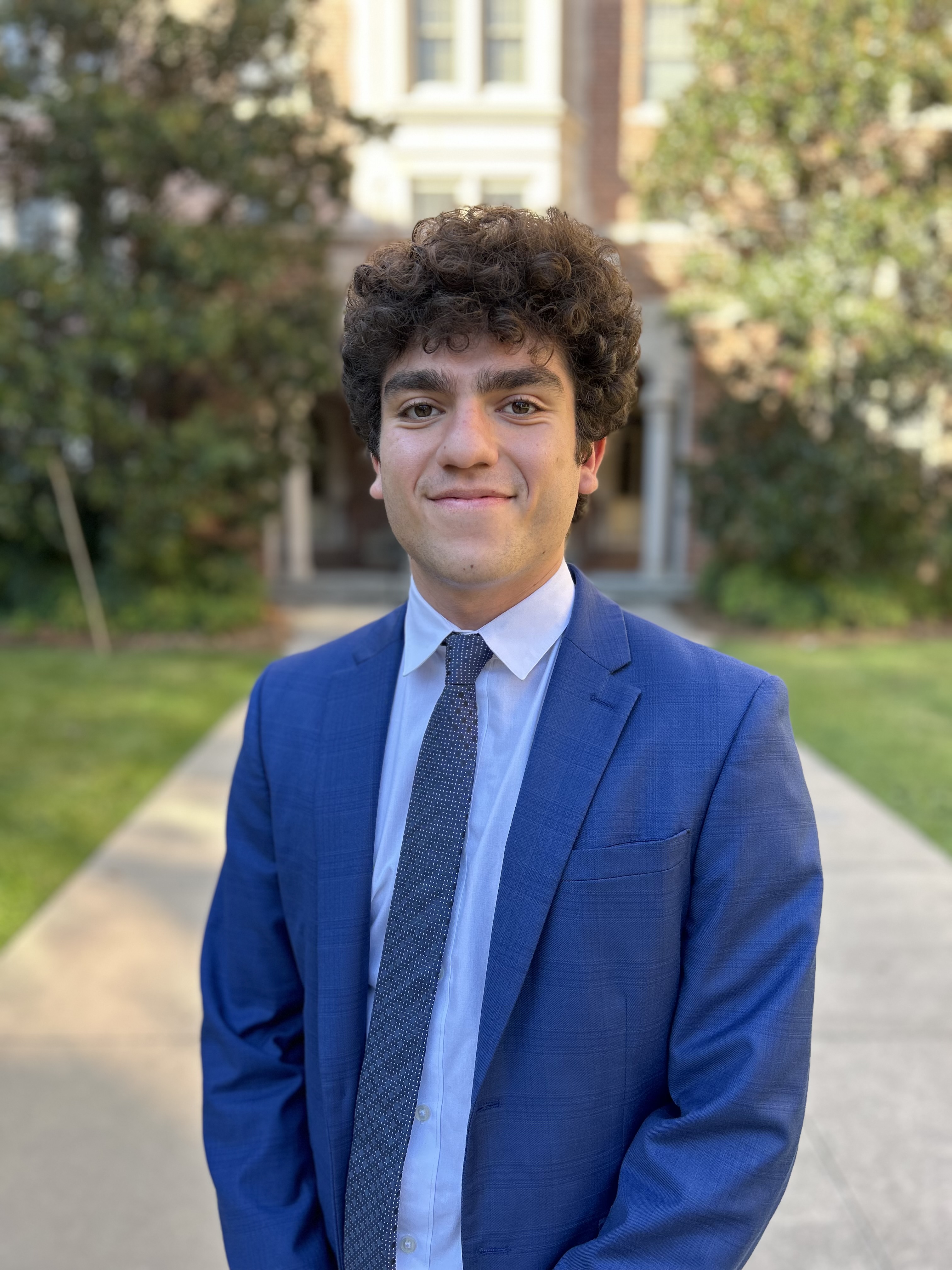
BIO
Samir Kanbar is a computer science major based in Miami with a passion for entrepreneurship and technology. As the founder of Komp, a social networking platform for pick-up sports athletes, he is dedicated to building community-driven solutions that enhance engagement and connectivity. Samir has a strong background in software development, spanning backend architecture, frontend design, and API integrations. He is actively involved in business development, networking with faculty, and seeking funding to scale his ventures. A Lebanese immigrant and Presidential Scholar at FSU, Samir is driven by a vision to make a lasting impact in the tech and startup space.
Enhancing Anomaly Detection: Innovative Approaches for Accurate and Efficient Detection
Authors: Samir Kanbar, Daniel SchwartzStudent Major: Computer Science
Mentor: Daniel Schwartz
Mentor's Department: Computer Science Mentor's College: College of Arts and Sciences Co-Presenters:
Abstract
Anomaly detection plays a crucial role in identifying irregular patterns across various domains, including cybersecurity, healthcare, and finance. This research explores innovative approaches to efficiently and accurately detect anomalies using machine learning, rule-based systems, and hybrid techniques. The study outlines a structured methodology encompassing data collection, preprocessing, and experimental setup, leveraging tools such as Python and TensorFlow. Performance metrics like accuracy, precision, and recall are analyzed through visual representations, providing insights into model effectiveness. Key limitations, including data constraints and model complexity, are discussed alongside future research directions to enhance detection capabilities. This work aims to contribute to the advancement of anomaly detection systems, paving the way for more reliable and scalable solutions in critical applications.
Keywords: Machine Learning, Anomaly Detection, Planning