Research Symposium
25th annual Undergraduate Research Symposium, April 1, 2025
Preetam Chamkura Poster Session 1: 9:30 am - 10:30 am/ Poster #202
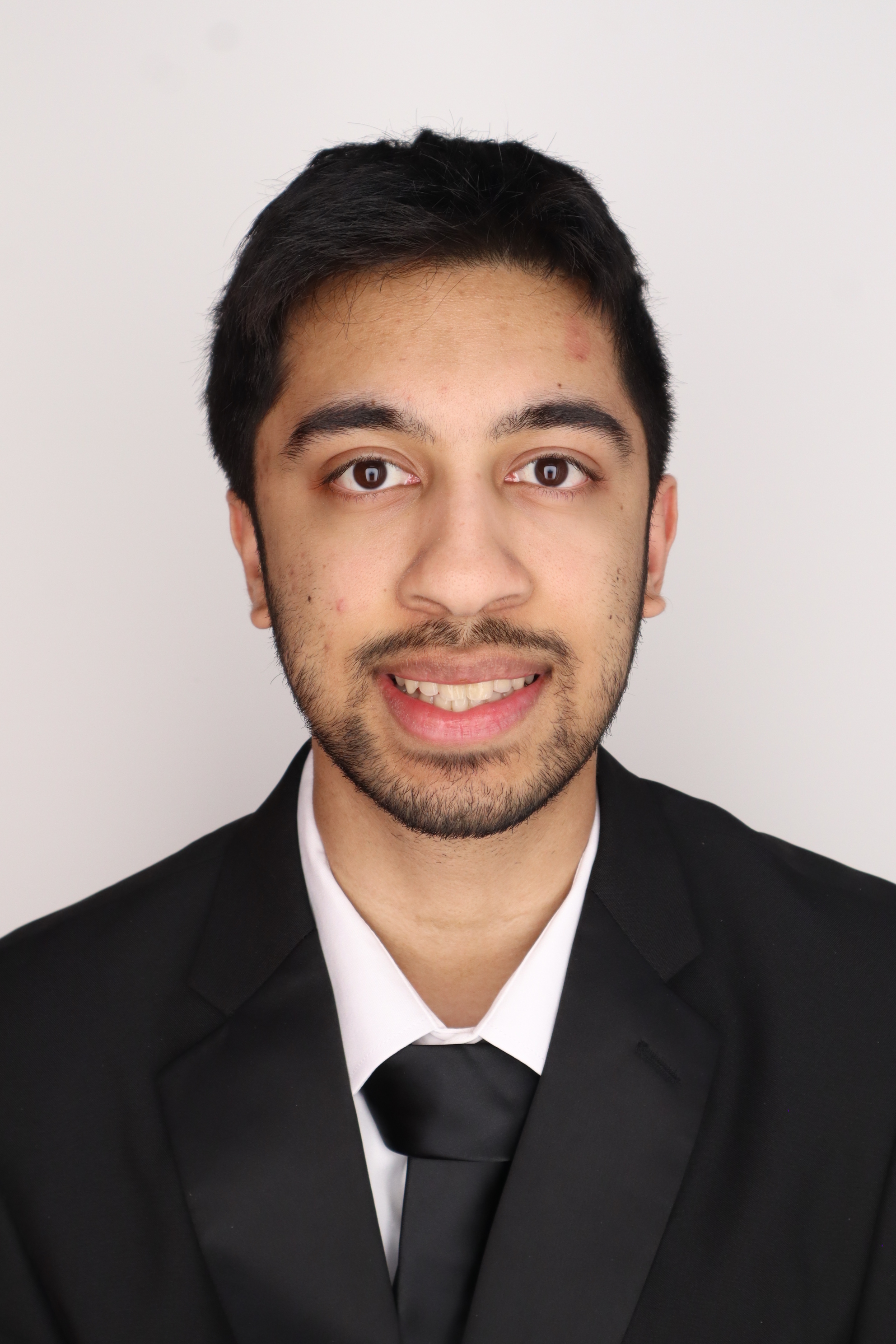
BIO
My name is Preetam Chamkura, and I'm a second-year Computer Science student here at FSU. I am interested in AI and how to use it to solve real-world issues. My career interests include machine learning, data analysis, and cybersecurity and how they can impact fields in the real world.
A Human-AI Collaborative Approach to Generate Tailored Questions about Lab Test Results
Authors: Preetam Chamkura, Dr. Zhe HeStudent Major: Computer Science
Mentor: Dr. Zhe He
Mentor's Department: School of Information Mentor's College: College of Communication and Information Co-Presenters:
Abstract
Patient portals can improve patient engagement and healthcare outcomes, especially for older adults with chronic conditions. However, limited health literacy and technology skills hinder their ability to interpret lab results effectively. This research aims to develop LabGenie, a web-based tool designed to enhance older adults’ comprehension of lab results.
We have been evaluating the feasibility of using generative large language models to generate lab results-related questions from patients' electronic health records. After we used GPT 4.0 to generate 40-60 questions for each of the three selected patients with diabetes and chronic kidney disease, we interviewed three physicians to gather insights into lab test selection, clinical relevance, and AI-driven question generation. Thematic analysis of the transcripts revealed five key themes: project planning and progress, data and lab tests, AI and technology, collaboration and feedback, and research considerations. These themes informed the iterative design of LabGenie’s AI-powered question generation module.
Preliminary findings suggest that focusing on standardized and symptom-driven lab test selection, while tailoring question prompts to individual patient needs, can improve usability and engagement. Physicians emphasized the importance of aligning lab test selection with older adults’ common conditions, such as diabetes and hypertension, and ensuring AI-generated questions are clinically relevant and actionable.
This study demonstrates the value of integrating clinician feedback and user-centered methodologies in digital health tool development. LabGenie has the potential to improve accessibility, patient engagement, and shared decision-making, ultimately enhancing health outcomes for older adults.
Keywords: AI, Health Literacy, Lab Result Interpretation, LLM, Healthcare