Research Symposium
24th annual Undergraduate Research Symposium, April 3, 2024
Pietro Candiani Poster Session 2: 10:45 am - 11:45 am/230
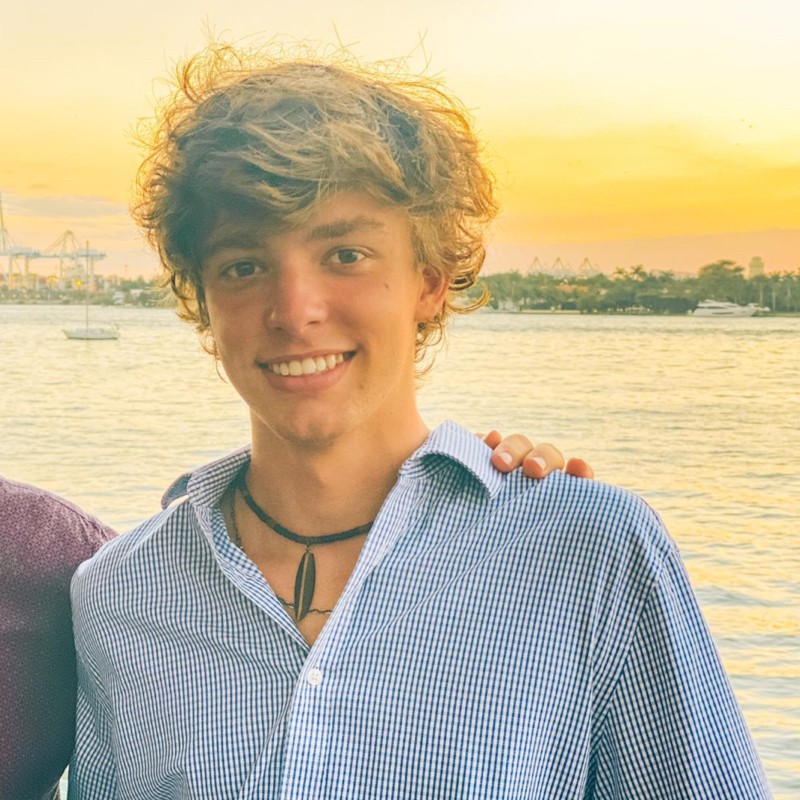
BIO
Interested in the application of machine learning to solver real world problems. Since I was little I was passionate to problem solving which has lead me t double major in computer science and pure math, and I hope to use this skills to help solve problems.
Applications of Machine Learning in Finance
Authors: Pietro Candiani, Arafatur RahmanStudent Major: Mathematics and Computer Science
Mentor: Arafatur Rahman
Mentor's Department: Financial Mathematics Mentor's College: Florida State University Co-Presenters: Alexander Khan
Abstract
This study explores the predictive capabilities of Long Short-Term Memory (LSTM) models in forecasting the stock price movements of the S&P 500. The model utilizes a comprehensive set of features, including historical stock prices, macroeconomic indicators, and technical indicators. By conducting a comparative analysis, this research assesses the performance of the models and the significance of different features. The findings reveal that the LSTM model's effectiveness varies with the inclusion of specific features and the chosen timeframes. Additionally, the study examines the impact of market volatility events, such as the 2008 financial crisis and the COVID-19 pandemic, on predictive accuracy. Highlighting the potential of technical indicators in market condition predictability, the project offers insights for future advancements in financial market forecasting models.
Keywords: Machine Learning, Deep Learning, Finance, Stock Prediction