Research Symposium
24th annual Undergraduate Research Symposium, April 3, 2024
Cameron Cook He/Him Poster Session 3: 1:30 pm - 2:30 pm /451
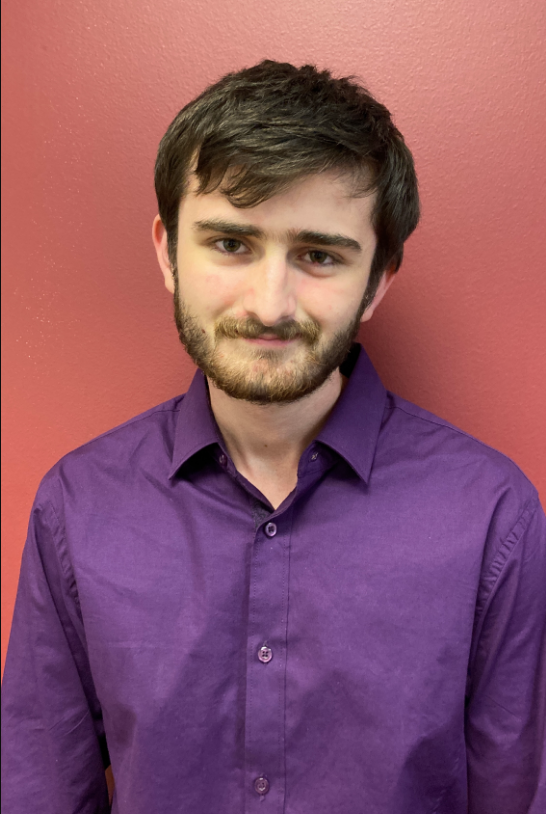
BIO
I'm a pre-meteorology student at Florida State University learning about the implications and solutions of meteorological events in every day life. I'm currently working to gain meteorological knowledge in every regard I can in order to make the greatest positive impact I can as a result regarding the global climate crisis.
Flood Data Collection And Processing For A Machine Learning Model
Authors: Cameron Cook, Maryam PakdehiStudent Major: Meteorology
Mentor: Maryam Pakdehi
Mentor's Department: Civil Engineering Mentor's College: Florida A&M University-Florida State University College of Engineering Co-Presenters:
Abstract
This research underscores the critical role of precise flood depth estimation in flood risk management and introduces a machine learning methodology tailored to enhance the accuracy of such estimations through hindcasting. The study primarily investigates the applicability and adaptability of a machine learning neural network model, initially developed for Hurricane Ida in the Lower Hudson watershed, by testing its performance on different hurricanes and locations. The goal is to evaluate the model's effectiveness across varying locations, conditions, and scenarios, and to understand the factors influencing the machine learning model’s performance.
To train and validate the machine learning algorithms, data from the NOAA and the National Hurricane Center were collected, filtered, and analyzed from nine hurricanes’ impacts between 2011 and 2022, each on one of six watersheds. These vital parameters included stream gauge water level, soil moisture, rainfall, tide, wind speed, and storm track data. This dataset was essential to train and test the machine learning model to grasp the complexities of flood dynamics and enhance its predictive capabilities.
While the results of this extensive study are pending, the anticipated outcomes aim to offer valuable insights into the viable efficacy of machine learning in predicting flood depths. This research is poised to contribute significantly to the field of flood risk management, highlighting the potential of advanced predictive models in ensuring community safety and resilience against the backdrop of increasingly frequent and severe rainfall events.
Keywords: Weather, Engineering, Machine Learning, Hindcasting, ANN