Research Symposium
24th annual Undergraduate Research Symposium, April 3, 2024
Annabelle Shen Poster Session 2: 10:45 am - 11:45 am/244
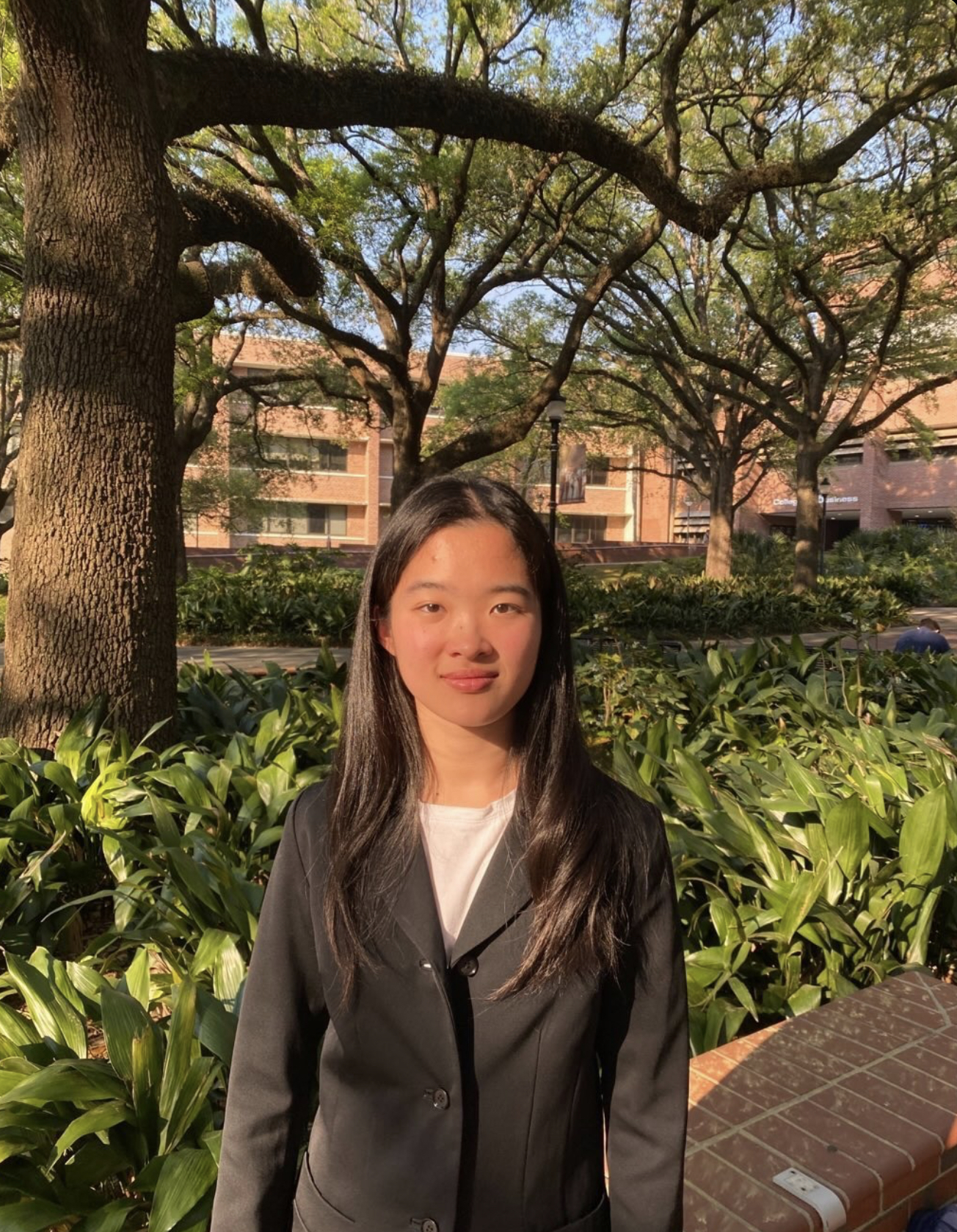
BIO
I am a freshman majoring in Chemistry at Florida State University. I have always been interested in discovering ways technology can improve our current healthcare systems. I hope to continue research in this area throughout college, and I aspire to be a physician in the future.
Providing Feedback for Surgical Training and Assessment Using Artificial Neural Networks
Authors: Annabelle Shen , Erim YanikStudent Major: Chemistry
Mentor: Erim Yanik
Mentor's Department: Engineering Mentor's College: FAMU-FSU College of Engineering Co-Presenters: Karah Martin
Abstract
Because current surgical residents are evaluated by attending physicians, this subjective, human nature can impact surgical outcomes, resulting in the loss of approximately 400,000 lives annually due to surgical errors. Through the use of artificial intelligence and computer vision, surgical tools can be classified and segmented, aiding in improving the objectivity and accuracy of real-time diagnoses. A Mask Region-based Convolutional Neural Networks (Mask RCNN will be used for object detection (classifying objects in images and videos) and instance segmentation (identifying different instances of the same object and separating the different objects through determining boundaries) respectively. Surgical frames will first be annotated using VGG image annotator. The pre-trained models will then be fine-tuned and trained on a specific surgical dataset to perform the specific task of tracking the movement of surgical tools in surgical recordings, which were collected from collaborations with surgeons in the Tallahassee, Florida area. Research is currently in progress; the deep learning model will go through training, validation, and eventually testing to obtain the model’s performance. This study represents a robust step towards integrating AI and CV in surgery, specifically via instance segmentation. While the results are pending, the potential for aiding in surgical diagnostics is promising.
Keywords: Deep learning, surgical evaluation, object detection