Research Symposium
24th annual Undergraduate Research Symposium, April 3, 2024
Caroline Bennett Poster Session 1: 9:30 am - 10:30 am /402
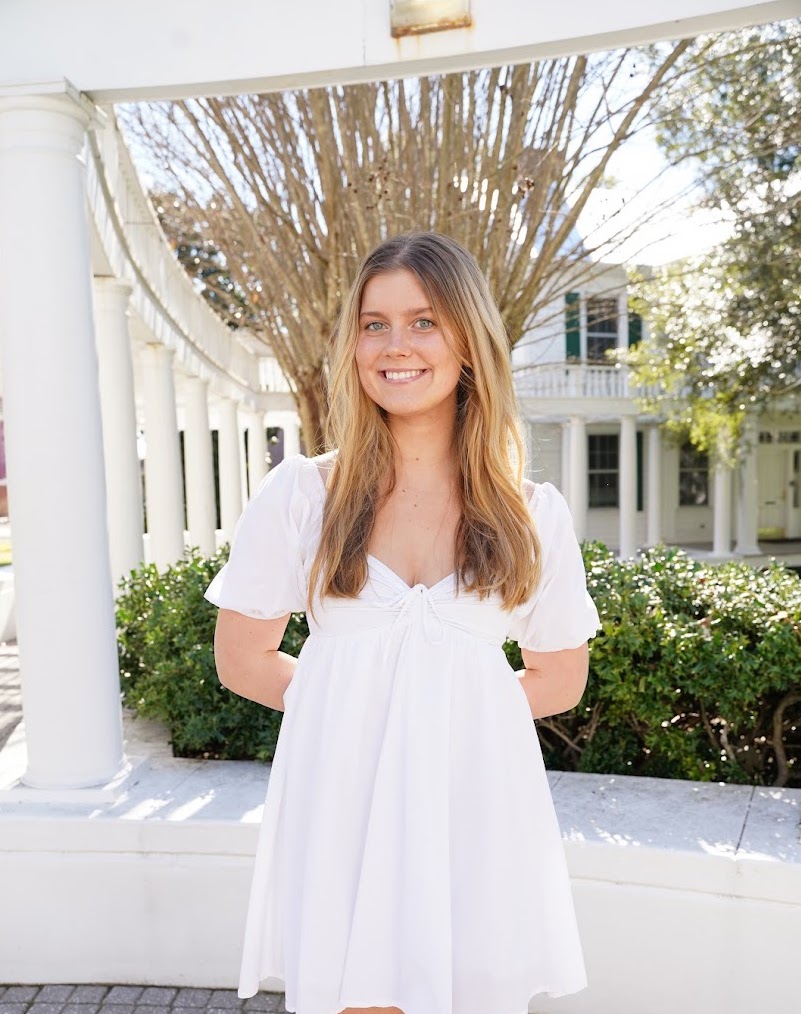
BIO
My name is Caroline Bennett and I am from Greenwich, Connecticut. I am currently pursuing a dual major in Cyber Criminology and Computer Science while on the pre-med track. I am passionate about technology, healthcare, and security, and my current research interests center on harnessing technology to revolutionize the health care world. Beyond my academic pursuits, I am thoroughly involved in the sorority Sigma Delta Tau as Philanthropy and Service Director and have grown to have a strong love for my Best Buddies family and the organization itself. Looking ahead, I envision a career at the intersection of medicine, technology, and security. My goal is to develop innovative solutions that safeguard sensitive healthcare data, contribute to a safer, more resilient healthcare system for all, and exercise my knowledge and skills to make a positive impact.
Evaluating Large Language Models for Accurate Lab Test Question Interpretation
Authors: Caroline Bennett, Zhe HeStudent Major: Cyber Criminology, Computer Science, pre-med track
Mentor: Zhe He
Mentor's Department: School of Information Mentor's College: College of Communication and Information Co-Presenters: Angelique Deville, Hailey Thompson
Abstract
The LabGenie project aims to address the challenge of patients, especially the elderly, in understanding medical lab test results and acting upon them. Even though generative AI models such as ChatGPT can answer questions, patients may not know what questions to ask, and they may also generate answers with inaccurate information or hallucinations. In the eHealth Lab, we are developing informatics strategies to augment large language models (LLM) by 1) identifying credible health sources for lab test result interpretation, and 2) curating these sources to computable format. As such, they can be used for question prompt enrichment with human input and retrieval-augmented generation (RAG). The ultimate goal is to integrate the RAG-based LLMs with a user-friendly interface for patients. LabGenie seeks to allow patients with low health literacy to ask contextualized questions and make informed health decisions with their providers confidently. The research involves literature reviews on LLM capabilities in clinical settings, converting lab result interpretation into a table format, and evaluate strengths and weaknesses of different LLMs in answering lab result questions. These procedures aim to provide meaningful results to train LLMs and contribute to the creation of LabGenie.
Keywords: AI, Health Literacy, Lab Result Interpretation