Research Symposium
24th annual Undergraduate Research Symposium, April 3, 2024
Marco Garza Poster Session 4: 2:45 pm - 3:45 pm /316
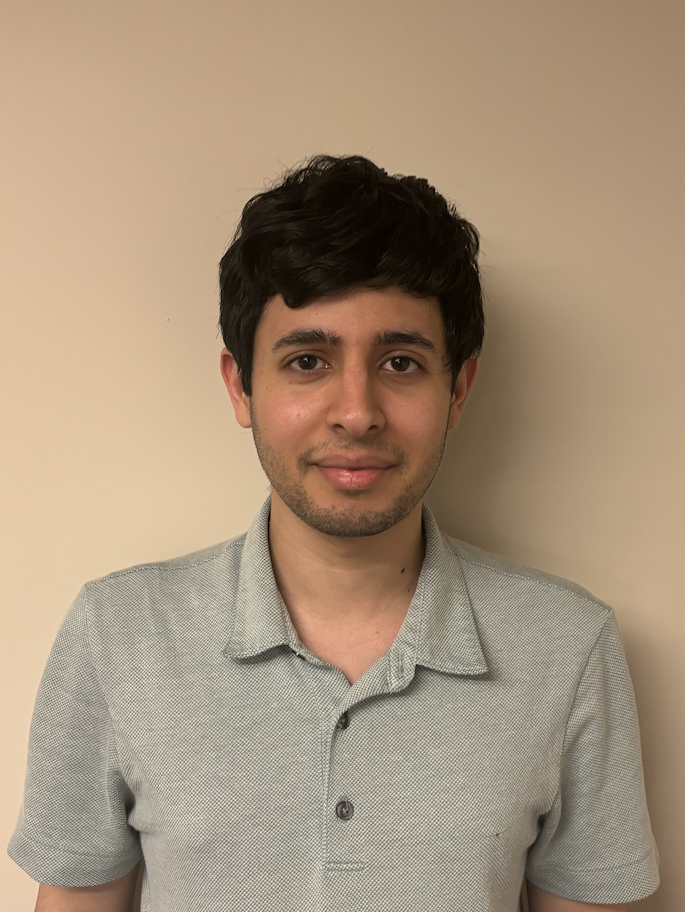
BIO
My name is Marco Garza and I am from Miami, Florida. I am passionate about human mechanisms and communicating ideas, as well as fostering, as well as fostering a learning environment. I plan to study mechanical engineering further to aid others through connecting the physical and human world.
Applications of Bayesian Analysis on Updating User Intent
Authors: Marco Garza, Dr. Taylor HigginsStudent Major: Mechanical Engineering
Mentor: Dr. Taylor Higgins
Mentor's Department: Department of Mechanical Engineering Mentor's College: University of Notre Dame (PhD, M.S) , Clemson University (B.S) Co-Presenters:
Abstract
To be beneficial, robotic lower-limb assistive robots need to able to infer what the user wants to do, this being considered the user's "intent". Previous intent recognition strategies have used external and onboard sensors on robotic devices and subjects to track force resistance and muscle activity to provide signals for the user’s intent. We believe that including information about the user’s proximity to objects, the uses of these objects to user or "affordances", and the user’s orientation should increase the accuracy of our intent recognition. Our early literature review suggested that Bayesian networks, mathematical models that use conditional probabilities to represent variable relations, can be used to improve belief of the user’s intent from including such information. Bayesian analysis is a mathematical tool that allows us to calculate how these new signals should update our belief of the user's intent. For this project, I have experimented with various designs of a Bayesian network with which to base our intent recognition algorithm. I tested these designs using a software called SamIam, which is a program that allows us to create Bayesian networks and adjust their variable sets and conditional probabilities. We determined that it is necessary to rely on discrete variables in this analysis, instead of continuous ones, to keep the computational time low. We are continuing to work to validate the utility of a particular Bayesian network design with real experimental data that includes information about the user's surroundings.
Keywords: Robotics, mathematical analysis, probability