Research Symposium
24th annual Undergraduate Research Symposium, April 3, 2024
Jesse Valdes Poster Session 2: 10:45 am - 11:45 am/306
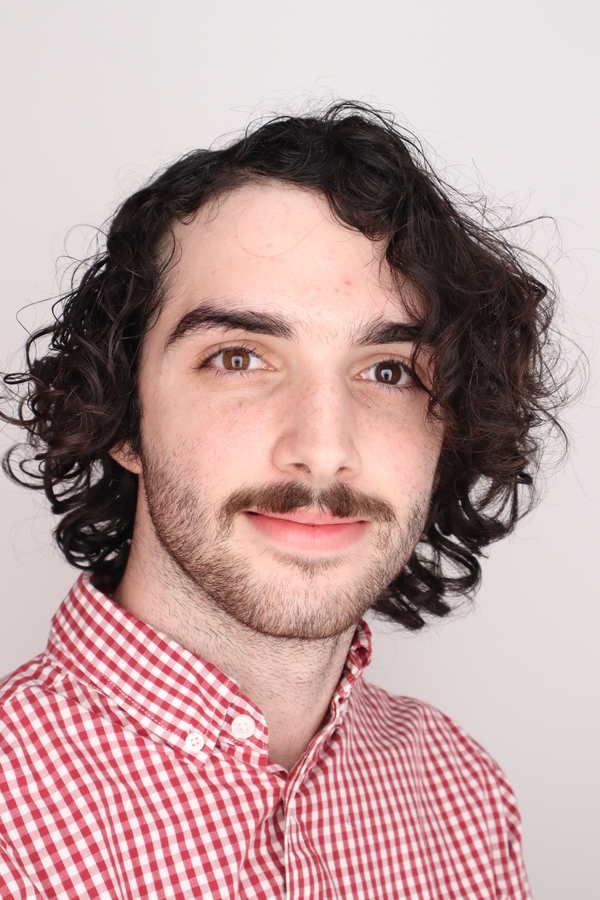
BIO
Jesse James Valdes specializes in economics and statistics with a passion for big data and its applications to societal challenges. With experience as a World Bank Group intern and as a research assistant, Jesse is adept in data collection and statistical analysis, particularly in classification. Committed to learning advanced data collection and analysis techniques, Jesse aspires to leverage big data insights for impactful decision-making in economics.
Construction of a Historical Infrastructure Price Index
Authors: Jesse Valdes, Dr. Carl KitchensStudent Major: Economics and Statistics
Mentor: Dr. Carl Kitchens
Mentor's Department: Economics Mentor's College: College of Social Sciences and Public Policy Co-Presenters:
Abstract
This research project aims to develop a comprehensive historical construction price index spanning from the 20th century onwards, recognizing significant shifts influenced by factors such as inflation, technological advancements, and efficiency improvements.
In the early 20th century, the pricing of American infrastructure construction lacked digitization. To address this, microfilms from the Engineering News-Record are digitized through scanning microfilm and processing to make them machine readable. Leveraging microfilm provides access to a historical journal with weekly editions dating back to the 19th century, enabling an examination of prices for various construction elements, job-related salaries, and awarded contracts. After processing the aggregate microfilm data, images are corrected for transcription errors, and weights are assigned to individual projects. The organized aggregate data is then categorized at the city-year-infrastructure type level.
The extraction process employs text parsing and image formatting techniques to unveil relevant construction pricing information. This involves identifying monthly awarded construction contracts based on regional parameters. Specifically, machine learning methods, including Amazon Textract and Python data scraping syntax, are utilized to efficiently extract construction pricing from thousands of pages at a time.
The findings from this project hold the potential to assist policymakers and those involved in constructing new buildings in estimating potential costs. By identifying trends among historical decisions, this information contributes to more informed decision-making regarding future construction expenses.
Keywords: Economics, OCR, Construction, Inflation, Infrastructure