Research Symposium
24th annual Undergraduate Research Symposium, April 3, 2024
Miles Rosoff Poster Session 4: 2:45 pm - 3:45 pm/60
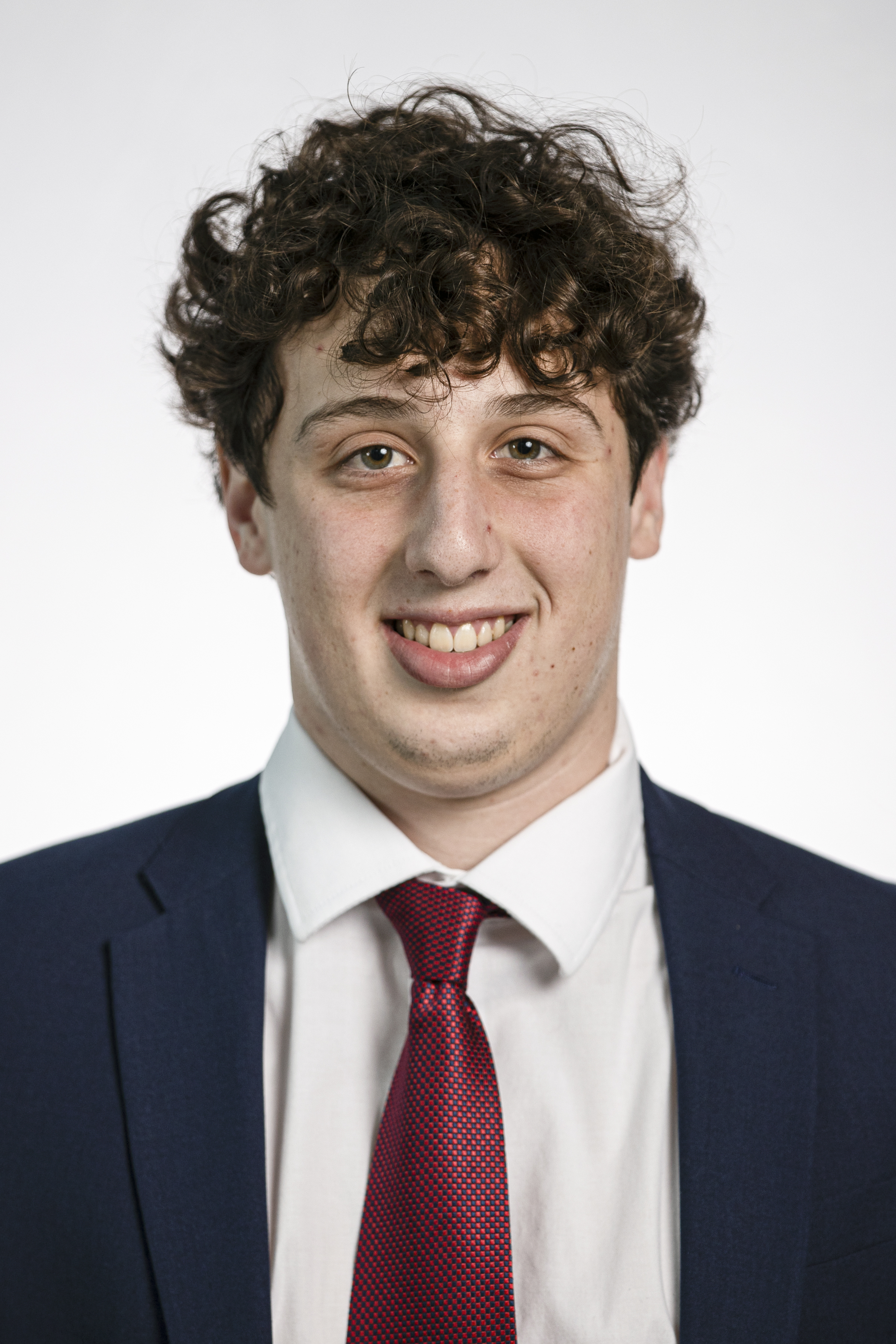
BIO
My name is Miles Rosoff, and I am a dedicated second-year undergraduate from Coral Springs, Florida, pursuing a major in Computational Science. With a keen interest in the cutting-edge fields of Artificial Intelligence and Large Language Models, I am particularly fascinated by exploring the most efficient methodologies for leveraging these technologies. My background in coding, with a strong emphasis on Python and C++, perfectly aligns with my academic interests, providing a strong foundation for my involvement in this research project.
Do Large Language Models Reason in a Bayesian Fashion?
Authors: Miles Rosoff, Gordon ErlebacherStudent Major: Computational Science
Mentor: Gordon Erlebacher
Mentor's Department: Computational Science Mentor's College: College of Arts and Sciences Co-Presenters: Thomas Cherry, Hoang Vu
Abstract
We investigate the hypothesis that large language models (LLMs) such as GPT-4, Mixtral-8x7B, and Phi-1.5 learn concepts in a manner consistent with Bayesian inference. To assess this capability, the LLMs are tasked with guessing a concept given a sequence of words. We first approximate the LLMs' prior over concepts to then approximate its posterior over concepts after a word has been presented to it. We then compare the LLM posterior with that from Bayesian inference. Additionally, the study explores the extent to which temperature influences the posterior's conformity to Bayes' Rule. Our investigation aims to enrich the understanding of Bayesian reasoning in LLMs and its implications for model performance. Our results suggest that the posterior update does not conform to Bayesian statistics, invalidating the original hypothesis.
Keywords: AI, LLM, Bayesian, Computational Science